Meet Jenny O'Brien
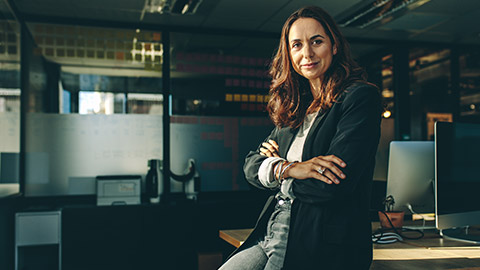
This is Jenny O'Brien from XYZ Manufacturing.
Jenny performs a leadership and management role at XYZ Manufacturing as a Business Operations Manager. Jenny is currently training to use big data for operational decision-making.
In this module, you will learn the skills and knowledge that enable someone like Jenny to adopt new, more efficient, data-driven ways to make operational decisions using big data analytics. This would involve knowing how to:
- apply skills in decision making
- apply techniques for using big data in day-to-day work
- analyse, interpret and apply insights from big data when making operational decisions.
The following video discusses the role of business leaders when using big data for operational decision-making in their organisations.
In this topic, you will learn the basic concepts of big data, the data-driven decision-making process and the new data analytic skills and techniques Jenny would need to acquire as a business leader.
What is big data?
Gartner's popular definition of big data is as follows.
Big data is high-volume, high-velocity and/or high-variety information assets that demand cost-effective, innovative forms of information processing that enable enhanced insight, decision making, and process automation. 1(Gartner 2022)
Organisations receive huge amounts of data from daily interactions and processes. Big data is more than just large amounts of data. It includes data of different types from different sources and in large volumes. Various sources such as social media platforms, weblogs, Internet of Things (IoT) devices such as sensors found in smart devices and many more produce massive amounts of data.
The five key characteristics that make big data useful are often referred to as the 5 Vs of big data.
- Volume refers to the amount of data large enough for the task at hand.
- Velocity refers to quick data capture and analysis for timely business decisions.
- Variety refers to related data from different data types and sources.
- Veracity refers to reliable and trustworthy data.
- Value refers to data analysis that provides value.
Raw data vs. datasets
Raw data refers to big data that is in its natural form and is unprocessed. This includes large amounts of data acquired from various big data sources.2
The term dataset refers to big data that is already transformed, cleaned and organised. 3
A dataset is more manageable and has gone through preliminary data-cleaning processes to be ready for further analysis. Analytical models can be run easily on big datasets to produce accurate findings and would improve the overall big data analysis process.
Also, note that datasets are derived from raw data and are often considered subsets or representative samples of raw data.
Data sources
An organisation can obtain big data from two main categories of sources. They are in-house sources and external sources.
Expand each item below to see more details.
In-house sources of big data are those generated, owned and controlled by the organisation.4
Some examples of in-house data sources include:
- Transactional data and point of sale (POS) information – which includes both current and historical data that relates to the organisations’ own purchases and details of their customers’ purchasing trends.
- Customer relationship management system (CRM) – this holds details of customers, which can be further analysed to understand where customers are located geographically and their company affiliations.
- In-house data systems – Enterprise resource planning (ERP), finance systems or other organisation databases.
- In-house documents – Today, in-house documents are mostly in Microsoft form (PDFs, Word Documents, Spreadsheets etc.), and they provide a wealth of information about business activities, policies and procedures.
- Archives – Historical data can contain a wealth of information on how the business used to operate and help further investigate patterns or to resolve issues.
- Device sensors – Any Internet of Things (IoT) device used within the icrosoft on can generate data on network connectivity as it relates to the business. For example, a transport company can use sensor data received from their vehicles to source valuable information such as milage, fuel consumption, travel route etc.
External sources of big data are those generated outside of the organisation.
Data obtained from external sources may include:
- public data (e.g. publicly available data for machine learning, official statistics, forecasts)
- unstructured data (e.g. social media posts)
- other types of data gathered by third-party organisations (e.g. Peak body data, Government data, data collected from Google).
An organisation may obtain permission to use these sources but does not own or control them.
Knowledge check
Complete the following five (5) questions. Click the arrows to navigate between the questions.
The value of big data lies in our ability to extract insights and make better decisions 5(Rappa 2012)
Big data plays a vital role in enabling businesses to make informed decisions. With recent technological improvements, harvesting and processing large volumes of data is now economical. Combining this with accurate analytics can yield a significant competitive advantage.
Benefits of making data-driven decisions
Today, big data analytics is commonly used by a broad range of industries, including small businesses, to drive operational decision-making.
The following video discusses various ways organisations can benefit from the data-driven decision-making approach. When watching the video pay close attention to keywords such as ‘data-driven decisions’ and ‘data analytics’.
After watching the video, answer the following questions.
Knowledge check
Complete the following four (4) questions. Click the arrows to navigate between the questions.
The data-driven decision-making process
The following video explains the process of incorporating data-driven decision-making into any business. It also highlights the need for those in leadership and management positions to be actively involved in this process.
The procedure for data-driven decision-making includes the following key steps.
- Gathering business requirements - involves clearly defining the problem to be solved, understanding the business objective, the available data, and the constraints and limitations of the problem.
- Accessing big data sources and summaries - involves researching the data sources and summaries relevant to the analysis. It is important to find out where to source the data and comply with legislative requirements, organisational policies and procedures to maintain data protection and privacy requirements of the data.
- Combining external and in-house data - involves gathering all necessary data sources and summaries (external and in-house) together into one place so that the combined data can be cleaned, formatted, and organised to create a dataset that can be used for the analysis.
- Analysing the data - involves analysing the dataset using statistical techniques to identify patterns and trends for developing insights into the problems.
- Integrating big data analytics into the operational workflow – involves developing reports that explore the impact of different decisions and outcomes. This type of analysis is commonly known as what-if analysis (see definition below), which uses simulation and optimisation tools to create scenarios that show how different decisions may impact business performance.
- Reporting on results and outcomes- involves reporting on the results from the what-if scenarios and evaluating which decision or outcome is most favourable. The scenarios are compared based on various criteria, such as cost, risk, and performance.
- Seeking input and feedback – involves obtaining input and feedback from the project's stakeholders. These stakeholders may be subject matter experts, management, supervisors and staff, who can provide feedback on the insights obtained from the report and confirm if it meets the operational decision-making requirements.
- Finalising and distributing the report – The final step is to use the insights gained from the analysis and what-if scenarios to make data-driven decisions. Finalising the report involves integrating the input and feedback received from the previous step. The final report also includes recommendations on the most favourable scenario that should be selected to make the relevant operational decisions.
A variety of policies and procedures apply to the entire process of using big data for operational decision-making, which we will further discuss in the next sub-topic.
Knowledge check
Complete the following five (5) questions. Click the arrows to navigate between the questions.
Industry examples
Finance industry
Operational decision-making is critical in the finance industry. Decisions made by financial institutions can have a significant impact on the economy and society. Big data can play an important role in helping financial institutions make data-driven operational decisions.
Using what-if scenarios, the bank can explore different lending strategies. For example, the bank can simulate the impact of different interest rates, repayment terms, and loan amounts to identify each customer segment's most effective lending strategy.
Based on the insights gained from big data analysis and what-if scenarios, the bank can make data-driven decisions that optimise its credit risk management and lending processes. This can result in reduced credit risk, improved profitability, and increased customer satisfaction.
The following video explains how the ‘National Australia Bank’ uses big data to improve customer service and make better financial decisions. Note the tools and platforms they use to conduct optimisations and simulations.
Retail industry
By analysing large amounts of sales data, retailers can identify patterns and trends in consumer behaviour to optimise inventory levels and reduce stockouts. For example, Walmart uses big data to optimise its inventory management, which has resulted in a significant reduction in out-of-stock items.6
Using what-if scenarios, retail companies can explore different strategies for managing their inventory levels. They can simulate the impact of different inventory policies, such as just-in-time inventory or safety stock, to identify the most effective strategy for each product category and location.
Retail companies can make data-driven decisions that optimise their inventory management and supply chain operations based on the insights gained from big data analysis and what-if scenarios. This can result in improved customer satisfaction, reduced costs, and increased profitability.
The following video explains how retail companies such as ‘Walmart’ uses big data to improve their business operations and better decision-making.
When making operational decisions using big data, getting the right data to the right people at the right time is vital.
Types of requirements
When using big data for operational decision-making, consider the following types of requirements.
- Data source requirements: Once you have a clear understanding of the business objectives and goals, the next step is to identify the key data sources that will be required to support the analysis. This may involve data from internal systems, external sources, or a combination of both. It is important to thoroughly review the available data and consult with subject matter experts to ensure the accuracy and relevance of the data used for the analysed.
- Timeline requirements: It is important to define the timelines for data processing and analysis, based on the business requirements. This may involve setting up a regular schedule for collecting and processing the data and defining the timeframes for delivering reports and other outputs.
- Decision-making requirements: Once you have identified the key data sources and defined the timelines, the next step is to identify the specific decision-making requirements that must be addressed. This may involve identifying key business metrics that will be used to measure success and determining the types of insights and recommendations that will be required to support decision-making.
- Reporting requirements: It is important to identify what type of report needs to be generated by the analysis (e.g. what-if analysis report, statistical analysis report and so on). Reporting requirements also include the format of the report, how it should be distributed, style guidelines to be applied, preferred visualisations to be used etc.
- Technology requirements: Finally, it is important to evaluate the technical requirements that will be needed to support the data processing and analysis. This may involve identifying the tools and technologies required to process and analyse the data and the skills and expertise needed to implement and use these tools effectively.
Insights from the industry
An analyst may need to consider other types of requirements based on the complexity of business operations, workflows and systems. Such as,
- Process requirements –that state the documented expectations, targets and specifications for business processes.
- Functional requirements – that explain how the system must work.
- Non-functional requirements – that explain how the system should perform.
- Content/User requirements - for discussing the use cases for a project.
Requirements identification process
Using the SMART methodology is a common approach to identifying business objectives and goals. By following the steps in this methodology, organisations can ensure that they can capture the requirements related to meeting the business objective and therefore, make informed decisions based on reliable data and insights.
Set SMART goals
SMART is an acronym for:
- Specific: Goals and requirements need to be specific. (To answer who, what, where?)
- Measurable: The goals need to be measurable to determine if they have been achieved.
- Achievable (action-oriented): The requirements need to be realistically achievable.
- Relevant: The results will be relevant to your initial requirements.
- Time-bound: The goals should be planned for completion within a set time frame.
Read the article How to make your goals achievable by mindtools.com for further information.
Knowledge check
Complete the following six(6) questions. Click the arrows to navigate between the questions.
Topic summary
Congratulations on completing your learning for this topic Business in Action – Using big data for operational decision making.
In this topic, you learnt the fundamental concepts related to:
- big data and datasets
- sources of big data (in-house and external)
- the data-driven decision making process
- requirements for operational decision-making.
Check your learning
The final activity for this topic is a set of questions that will help you prepare for your formal assessment.
Knowledge check
Complete the following five (5) questions. Click the arrows to navigate between the questions.
What's next?
Next, we will learn about specific requirements for using big data in a business.
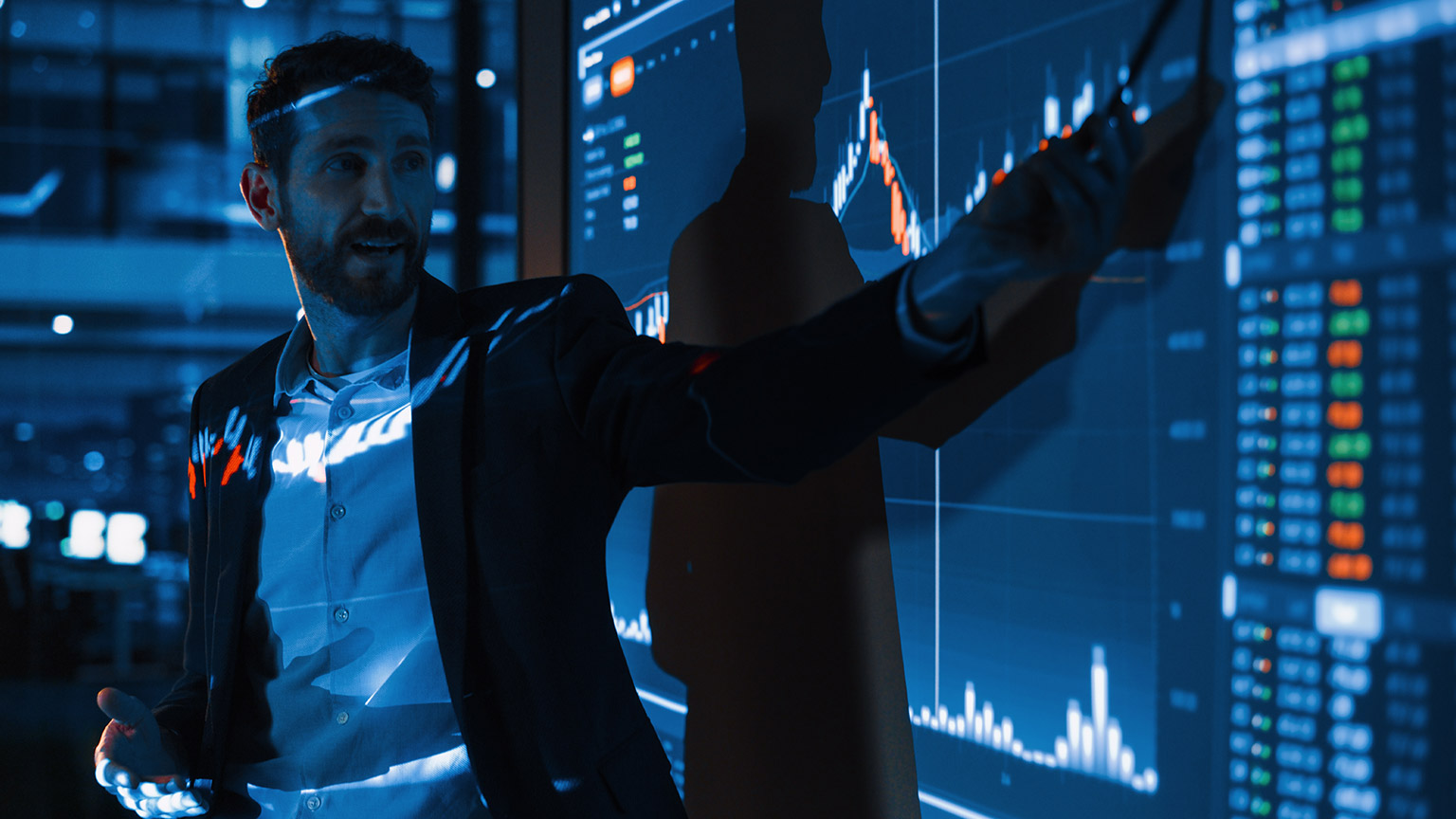